In Fall 2021, Harish Krishnakumar, then a junior at Tesla STEM high school in nearby Redmond, Washington, reached out to DiRAC Research Scientist Bryce Kalmbach to be his research mentor for a science fair project detecting and studying the properties of ring galaxies in large extragalactic galaxy surveys. Harish’s work eventually took him to the 2022 Regeneron International Science and Engineering Fair where he won two special awards during the competition.
Recently, Harish and Bryce have been working on a paper summarizing their work that has been submitted to the Astronomical Journal and is available on the ArXiv: https://arxiv.org/pdf/2210.11428.pdf.
The work presented in the paper uses convolutional neural networks (CNNs) to identify ring galaxies in postage stamp cutouts of galaxies from the Pan-Starrs 1 survey. CNNs are a commonly used deep learning method for classifying objects in large data sets of images. Since ring galaxies are a rare type of galaxy, one of the challenges of this work was finding enough ring galaxies to train the CNNs. For this, the authors turned to the Galaxy Zoo project which used human volunteers over many months to manually classify galaxy morphological types in images. They also used a technique called transfer learning where the neural networks were trained on a larger set of simulated galaxies before parts of the network were retrained on real data. Understanding and applying computational methods such as CNNs will be necessary to detect ring galaxies and other rare galaxy morphologies in the data provided by existing and future surveys like the Vera Rubin Observatory’s Legacy Survey of Space and Time (LSST). In the end, Harish and Bryce identified 1,151 new ring galaxies after examining over 900,000 galaxies in 10 hours of computation time showcasing a powerful new technique for finding rare morphologies of galaxies in large galaxy surveys.
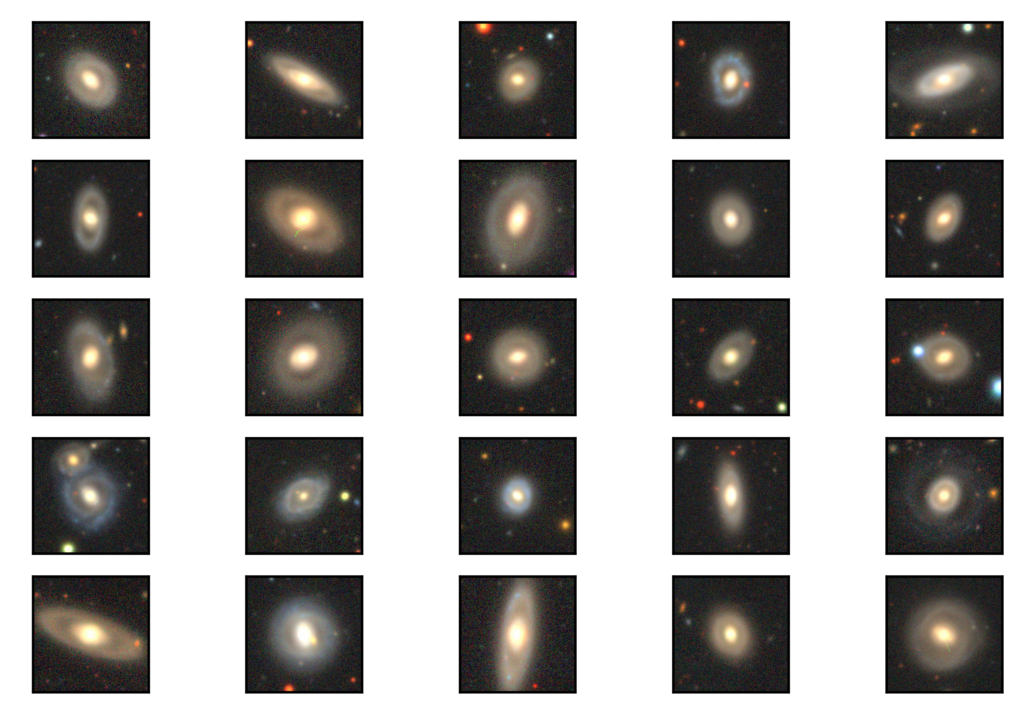